Better Data Quality Through Observability With Monte Carlo
Data Engineering Podcast - A podcast by Tobias Macey - Duminică
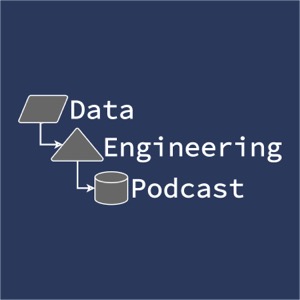
Categories:
Summary In order for analytics and machine learning projects to be useful, they require a high degree of data quality. To ensure that your pipelines are healthy you need a way to make them observable. In this episode Barr Moses and Lior Gavish, co-founders of Monte Carlo, share the leading causes of what they refer to as data downtime and how it manifests. They also discuss methods for gaining visibility into the flow of data through your infrastructure, how to diagnose and prevent potential problems, and what they are building at Monte Carlo to help you maintain your data’s uptime. Announcements Hello and welcome to the Data Engineering Podcast, the show about modern data management What are the pieces of advice that you wish you had received early in your career of data engineering? If you hand a book to a new data engineer, what wisdom would you add to it? I’m working with O’Reilly on a project to collect the 97 things that every data engineer should know, and I need your help. Go to dataengineeringpodcast.com/97things to add your voice and share your hard-earned expertise. When you’re ready to build your next pipeline, or want to test out the projects you hear about on the show, you’ll need somewhere to deploy it, so check out our friends at Linode. With their managed Kubernetes platform it’s now even easier to deploy and scale your workflows, or try out the latest Helm charts from tools like Pulsar and Pachyderm. With simple pricing, fast networking, object storage, and worldwide data centers, you’ve got everything you need to run a bulletproof data platform. Go to dataengineeringpodcast.com/linode today and get a $60 credit to try out a Kubernetes cluster of your own. And don’t forget to thank them for their continued support of this show! Are you bogged down by having to manually manage data access controls, repeatedly move and copy data, and create audit reports to prove compliance? How much time could you save if those tasks were automated across your cloud platforms? Immuta is an automated data governance solution that enables safe and easy data analytics in the cloud. Our comprehensive data-level security, auditing and de-identification features eliminate the need for time-consuming manual processes and our focus on data and compliance team collaboration empowers you to deliver quick and valuable data analytics on the most sensitive data to unlock the full potential of your cloud data platforms. Learn how we streamline and accelerate manual processes to help you derive real results from your data at dataengineeringpodcast.com/immuta. Today’s episode of the Data Engineering Podcast is sponsored by Datadog, a SaaS-based monitoring and analytics platform for cloud-scale infrastructure, applications, logs, and more. Datadog uses machine-learning based algorithms to detect errors and anomalies across your entire stack—which reduces the time it takes to detect and address outages and helps promote collaboration between Data Engineering, Operations, and the rest of the company. Go to dataengineeringpodcast.com/datadog today to start your free 14 day trial. If you start a trial and install Datadog’s agent, Datadog will send you a free T-shirt. You listen to this show to learn and stay up to date with what’s happening in databases, streaming platforms, big data, and everything else you need to know about modern data platforms. For more opportunities to stay up to date, gain new skills, and learn from your peers there are a growing number of virtual events that you can attend from the comfort and safety of your home. Go to dataengineeringpodcast.com/conferences to check out the upcoming events being offered by our partners and get registered today! Your host is Tobias Macey and today I’m interviewing Barr Moses and Lior Gavish about observability for your data pipelines and how they are addressing it at Monte Carlo. Interview Introduction How did you get involved in the area of data management? How did you come up with the idea to found Monte Carlo? What is "data downtime"? Can you start by giving your definition of observability in the context of data workflows? What are some of the contributing factors that lead to poor data quality at the different stages of the lifecycle? Monitoring and observability of infrastructure and software applications is a well understood problem. In what ways does observability of data applications differ from "traditional" software systems? What are some of the metrics or signals that we should be looking at to identify problems in our data applications? Why is this the year that so many companies are working to address the issue of data quality and observability? How are you addressing the challenge of bringing observability to data platforms at Monte Carlo? What are the areas of integration that you are targeting and how did you identify where to prioritize your efforts? For someone who is using Monte Carlo, how does the platform help them to identify and resolve issues in their data? What stage of the data lifecycle have you found to be the biggest contributor to downtime and quality issues? What are the most challenging systems, platforms, or tool chains to gain visibility into? What are some of the most interesting, innovative, or unexpected ways that you have seen teams address their observability needs? What are the most interesting, unexpected, or challenging lessons that you have learned while building the business and technology of Monte Carlo? What are the alternatives to Monte Carlo? What do you have planned for the future of the platform? Contact Info Visit www.montecarlodata.com?utm_source=rss&utm_medium=rss to lean more about our data reliability platform; Or reach out directly to [email protected] — happy to chat about all things data! Parting Question From your perspective, what is the biggest gap in the tooling or technology for data management today? Links Monte Carlo Monte Carlo Platform Observability Gainsight Barracuda Networks DevOps New Relic Datadog Netflix RAD Outlier Detection The intro and outro music is from The Hug by The Freak Fandango Orchestra / CC BY-SA Support Data Engineering Podcast