Build Your Python Data Processing Your Way And Run It Anywhere With Fugue
Data Engineering Podcast - A podcast by Tobias Macey - Duminică
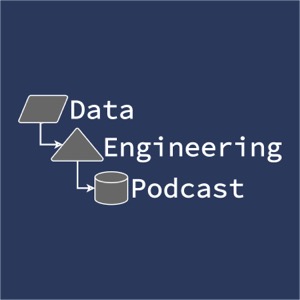
Categories:
Summary Python has grown to be one of the top languages used for all aspects of data, from collection and cleaning, to analysis and machine learning. Along with that growth has come an explosion of tools and engines that help power these workflows, which introduces a great deal of complexity when scaling from single machines and exploratory development to massively parallel distributed computation. In answer to that challenge the Fugue project offers an interface to automatically translate across Pandas, Spark, and Dask execution environments without having to modify your logic. In this episode core contributor Kevin Kho explains how the slight differences in the underlying engines can lead to big problems, how Fugue works to hide those differences from the developer, and how you can start using it in your own work today. Announcements Hello and welcome to the Data Engineering Podcast, the show about modern data management When you’re ready to build your next pipeline, or want to test out the projects you hear about on the show, you’ll need somewhere to deploy it, so check out our friends at Linode. With their managed Kubernetes platform it’s now even easier to deploy and scale your workflows, or try out the latest Helm charts from tools like Pulsar and Pachyderm. With simple pricing, fast networking, object storage, and worldwide data centers, you’ve got everything you need to run a bulletproof data platform. Go to dataengineeringpodcast.com/linode today and get a $100 credit to try out a Kubernetes cluster of your own. And don’t forget to thank them for their continued support of this show! Atlan is a collaborative workspace for data-driven teams, like Github for engineering or Figma for design teams. By acting as a virtual hub for data assets ranging from tables and dashboards to SQL snippets & code, Atlan enables teams to create a single source of truth for all their data assets, and collaborate across the modern data stack through deep integrations with tools like Snowflake, Slack, Looker and more. Go to dataengineeringpodcast.com/atlan today and sign up for a free trial. If you’re a data engineering podcast listener, you get credits worth $3000 on an annual subscription The only thing worse than having bad data is not knowing that you have it. With Bigeye’s data observability platform, if there is an issue with your data or data pipelines you’ll know right away and can get it fixed before the business is impacted. Bigeye let’s data teams measure, improve, and communicate the quality of your data to company stakeholders. With complete API access, a user-friendly interface, and automated yet flexible alerting, you’ve got everything you need to establish and maintain trust in your data. Go to dataengineeringpodcast.com/bigeye today to sign up and start trusting your analyses. Every data project starts with collecting the information that will provide answers to your questions or inputs to your models. The web is the largest trove of information on the planet and Oxylabs helps you unlock its potential. With the Oxylabs scraper APIs you can extract data from even javascript heavy websites. Combined with their residential proxies you can be sure that you’ll have reliable and high quality data whenever you need it. Go to dataengineeringpodcast.com/oxylabs today and use code DEP25 to get your special discount on residential proxies. Your host is Tobias Macey and today I’m interviewing Kevin Kho about Fugue, a library that offers a unified interface for distributed computing that lets users execute Python, pandas, and SQL code on Spark and Dask without rewrites Interview Introduction How did you get involved in the area of data management? Can you describe what Fugue is and the story behind it? What are the core goals of the Fugue project? Who are the target users for Fugue and how does that influence the feature priorities and API design? How does Fugue compare to projects such as Modin, etc. for abstracting over the execution engine? What are some of the sharp edges that contribute to the engineering effort required to migrate from a single machine to Spark or Dask? What are some of the determining factors that will influence the decision of whether to use Pandas, Spark, or Dask? Can you describe how Fugue is implemented? How have the design and goals of the project changed or evolved since you started working on it? How do you ensure the consistency of logic across execution engines? Can you describe the workflow of integrating Fugue into an existing or greenfield project? How have you approached the work of automating logic optimization across execution contexts? What are some of the risks or error conditions that you have to guard against? How do you manage validation of those optimizations, particularly as the different engines release new versions or capabilities? What are the most interesting, innovative, or unexpected ways that you have seen Fugue used? What are the most interesting, unexpected, or challenging lessons that you have learned while working on Fugue? When is Fugue the wrong choice? What do you have planned for the future of Fugue? Contact Info LinkedIn Email Fugue Twitter Parting Question From your perspective, what is the biggest gap in the tooling or technology for data management today? Closing Announcements Thank you for listening! Don’t forget to check out our other show, Podcast.__init__ to learn about the Python language, its community, and the innovative ways it is being used. Visit the site to subscribe to the show, sign up for the mailing list, and read the show notes. If you’ve learned something or tried out a project from the show then tell us about it! Email [email protected]) with your story. To help other people find the show please leave a review on iTunes and tell your friends and co-workers Links Fugue Fugue Tutorials Prefect Podcast Episode Bodo Podcast Episode Pandas DuckDB Koalas Dask Podcast Episode Spark Modin Podcast.__init__ Episode Fugue SQL Flink PyCaret ANTLR OmniSci Ibis The intro and outro music is from The Hug by The Freak Fandango Orchestra / CC BY-SA Support Data Engineering Podcast