Doing DataOps For External Data Sources As A Service at Demyst
Data Engineering Podcast - A podcast by Tobias Macey - Duminică
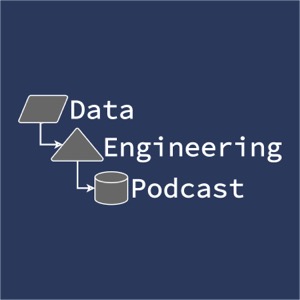
Categories:
Summary The data that you have access to affects the questions that you can answer. By using external data sources you can drastically increase the range of analysis that is available to your organization. The challenge comes in all of the operational aspects of finding, accessing, organizing, and serving that data. In this episode Mark Hookey discusses how he and his team at Demyst do all of the DataOps for external data sources so that you don’t have to, including the systems necessary to organize and catalog the various collections that they host, the various serving layers to provide query interfaces that match your platform, and the utility of having a single place to access a multitude of information. If you are having trouble answering questions for your business with the data that you generate and collect internally, then it is definitely worthwhile to explore the information available from external sources. Announcements Hello and welcome to the Data Engineering Podcast, the show about modern data management When you’re ready to build your next pipeline, or want to test out the projects you hear about on the show, you’ll need somewhere to deploy it, so check out our friends at Linode. With their managed Kubernetes platform it’s now even easier to deploy and scale your workflows, or try out the latest Helm charts from tools like Pulsar and Pachyderm. With simple pricing, fast networking, object storage, and worldwide data centers, you’ve got everything you need to run a bulletproof data platform. Go to dataengineeringpodcast.com/linode today and get a $100 credit to try out a Kubernetes cluster of your own. And don’t forget to thank them for their continued support of this show! Struggling with broken pipelines? Stale dashboards? Missing data? If this resonates with you, you’re not alone. Data engineers struggling with unreliable data need look no further than Monte Carlo, the world’s first end-to-end, fully automated Data Observability Platform! In the same way that application performance monitoring ensures reliable software and keeps application downtime at bay, Monte Carlo solves the costly problem of broken data pipelines. Monte Carlo monitors and alerts for data issues across your data warehouses, data lakes, ETL, and business intelligence, reducing time to detection and resolution from weeks or days to just minutes. Start trusting your data with Monte Carlo today! Visit dataengineeringpodcast.com/montecarlo to learn more. The first 10 people to request a personalized product tour will receive an exclusive Monte Carlo Swag box. Are you bored with writing scripts to move data into SaaS tools like Salesforce, Marketo, or Facebook Ads? Hightouch is the easiest way to sync data into the platforms that your business teams rely on. The data you’re looking for is already in your data warehouse and BI tools. Connect your warehouse to Hightouch, paste a SQL query, and use their visual mapper to specify how data should appear in your SaaS systems. No more scripts, just SQL. Supercharge your business teams with customer data using Hightouch for Reverse ETL today. Get started for free at dataengineeringpodcast.com/hightouch. Your host is Tobias Macey and today I’m interviewing Mark Hookey about Demyst Data, a platform for operationalizing external data Interview Introduction How did you get involved in the area of data management? Can you describe what Demyst is and the story behind it? What are the services and systems that you provide for organizations to incorporate external sources in their data workflows? Who are your target customers? What are some examples of data sets that an organization might want to use in their analytics? How are these different from SaaS data that an organization might integrate with tools such as Stitcher and Fivetran? What are some of the challenges that are introduced by working with these external data sets? If an organization isn’t using Demyst what are some of the technical and organizational systems that they will need to build and manage? Can you describe how the Demyst platform is architected? What have been the most complex or difficult engineering challenges that you have dealt with while building Demyst? Given the wide variance in the systems that your customers are running, what are some strategies that you have used to provide flexible APIs for accessing the underlying information? What is the process for you to identify and onboard a new data source in your platform? What are some of the additional analytical systems that you have to run to manage your business (e.g. usage metering and analytics, etc.)? What are the most interesting, innovative, or unexpected ways that you have seen Demyst used? What are the most interesting, unexpected, or challenging lessons that you have learned while working on Demyst? When is Demyst the wrong choice? What do you have planned for the future of Demyst? Contact Info LinkedIn Email Parting Question From your perspective, what is the biggest gap in the tooling or technology for data management today? Closing Announcements Thank you for listening! Don’t forget to check out our other show, Podcast.__init__ to learn about the Python language, its community, and the innovative ways it is being used. Visit the site to subscribe to the show, sign up for the mailing list, and read the show notes. If you’ve learned something or tried out a project from the show then tell us about it! Email [email protected]) with your story. To help other people find the show please leave a review on iTunes and tell your friends and co-workers Links Demyst Data LexisNexis AWS Athena DataRobot The intro and outro music is from The Hug by The Freak Fandango Orchestra / CC BY-SA Support Data Engineering Podcast