Easily Build Advanced Similarity Search With The Pinecone Vector Database
Data Engineering Podcast - A podcast by Tobias Macey - Duminică
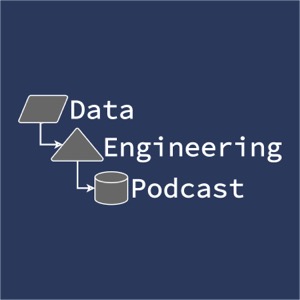
Categories:
Summary Machine learning models use vectors as the natural mechanism for representing their internal state. The problem is that in order for the models to integrate with external systems their internal state has to be translated into a lower dimension. To eliminate this impedance mismatch Edo Liberty founded Pinecone to build database that works natively with vectors. In this episode he explains how this technology will allow teams to accelerate the speed of innovation, how vectors make it possible to build more advanced search functionality, and how Pinecone is architected. This is an interesting conversation about how reconsidering the architecture of your systems can unlock impressive new capabilities. Announcements Hello and welcome to the Data Engineering Podcast, the show about modern data management When you’re ready to build your next pipeline, or want to test out the projects you hear about on the show, you’ll need somewhere to deploy it, so check out our friends at Linode. With their managed Kubernetes platform it’s now even easier to deploy and scale your workflows, or try out the latest Helm charts from tools like Pulsar and Pachyderm. With simple pricing, fast networking, object storage, and worldwide data centers, you’ve got everything you need to run a bulletproof data platform. Go to dataengineeringpodcast.com/linode today and get a $100 credit to try out a Kubernetes cluster of your own. And don’t forget to thank them for their continued support of this show! RudderStack’s smart customer data pipeline is warehouse-first. It builds your customer data warehouse and your identity graph on your data warehouse, with support for Snowflake, Google BigQuery, Amazon Redshift, and more. Their SDKs and plugins make event streaming easy, and their integrations with cloud applications like Salesforce and ZenDesk help you go beyond event streaming. With RudderStack you can use all of your customer data to answer more difficult questions and then send those insights to your whole customer data stack. Sign up free at dataengineeringpodcast.com/rudder today. When it comes to serving data for AI and ML projects, do you feel like you have to rebuild the plane while you’re flying it across the ocean? Molecula is an enterprise feature store that operationalizes advanced analytics and AI in a format designed for massive machine-scale projects without having to manage endless one-off information requests. With Molecula, data engineers manage one single feature store that serves the entire organization with millisecond query performance whether in the cloud or at your data center. And since it is implemented as an overlay, Molecula doesn’t disrupt legacy systems. High-growth startups use Molecula’s feature store because of its unprecedented speed, cost savings, and simplified access to all enterprise data. From feature extraction to model training to production, the Molecula feature store provides continuously updated feature access, reuse, and sharing without the need to pre-process data. If you need to deliver unprecedented speed, cost savings, and simplified access to large scale, real-time data, visit dataengineeringpodcast.com/molecula and request a demo. Mention that you’re a Data Engineering Podcast listener, and they’ll send you a free t-shirt. Your host is Tobias Macey and today I’m interviewing Edo Liberty about Pinecone, a vector database for powering machine learning and similarity search Interview Introduction How did you get involved in the area of data management? Can you start by describing what Pinecone is and the story behind it? What are some of the contexts where someone would want to perform a similarity search? What are the considerations that someone should be aware of when deciding between Pinecone and Solr/Lucene for a search oriented use case? What are some of the other use cases that Pinecone enables? In the absence of Pinecone, what kinds of systems and solutions are people building to address those use cases? Where does Pinecone sit in the lifecycle of data and how does it integrate with the broader data management ecosystem? What are some of the systems, tools, or frameworks that Pinecone might replace? How is Pinecone implemented? How has the architecture evolved since you first began working on it? What are the most complex or difficult aspects of building Pinecone? Who is your target user and how does that inform the user experience design and product development priorities? For someone who wants to start using Pinecone, what is involved in populating it with data building an analysis or service with it? What are some of the data modeling considerations when building a set of vectors in Pinecone? What are some of the most interesting, unexpected, or innovative ways that you have seen Pinecone used? What are the most interesting, unexpected, or challenging lessons that you have learned while building and growing the Pinecone technology and business? When is Pinecone the wrong choice? What do you have planned for the future of Pinecone? Contact Info Website LinkedIn Parting Question From your perspective, what is the biggest gap in the tooling or technology for data management today? Closing Announcements Thank you for listening! Don’t forget to check out our other show, Podcast.__init__ to learn about the Python language, its community, and the innovative ways it is being used. Visit the site to subscribe to the show, sign up for the mailing list, and read the show notes. If you’ve learned something or tried out a project from the show then tell us about it! Email [email protected]) with your story. To help other people find the show please leave a review on iTunes and tell your friends and co-workers Join the community in the new Zulip chat workspace at dataengineeringpodcast.com/chat Links Pinecone Theoretical Physics High Dimensional Geometry AWS Sagemaker Visual Cortex Temporal Lobe Inverted Index Elasticsearch Podcast Episode Solr Lucene NMSLib Johnson-Lindenstrauss Lemma The intro and outro music is from The Hug by The Freak Fandango Orchestra / CC BY-SA Support Data Engineering Podcast