Effective Pandas Patterns For Data Engineering
Data Engineering Podcast - A podcast by Tobias Macey - Duminică
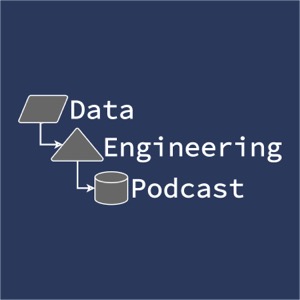
Categories:
Summary Pandas is a powerful tool for cleaning, transforming, manipulating, or enriching data, among many other potential uses. As a result it has become a standard tool for data engineers for a wide range of applications. Matt Harrison is a Python expert with a long history of working with data who now spends his time on consulting and training. He recently wrote a book on effective patterns for Pandas code, and in this episode he shares advice on how to write efficient data processing routines that will scale with your data volumes, while being understandable and maintainable. Announcements Hello and welcome to the Data Engineering Podcast, the show about modern data management When you’re ready to build your next pipeline, or want to test out the projects you hear about on the show, you’ll need somewhere to deploy it, so check out our friends at Linode. With their managed Kubernetes platform it’s now even easier to deploy and scale your workflows, or try out the latest Helm charts from tools like Pulsar and Pachyderm. With simple pricing, fast networking, object storage, and worldwide data centers, you’ve got everything you need to run a bulletproof data platform. Go to dataengineeringpodcast.com/linode today and get a $100 credit to try out a Kubernetes cluster of your own. And don’t forget to thank them for their continued support of this show! Today’s episode is Sponsored by Prophecy.io – the low-code data engineering platform for the cloud. Prophecy provides an easy-to-use visual interface to design & deploy data pipelines on Apache Spark & Apache Airflow. Now all the data users can use software engineering best practices – git, tests and continuous deployment with a simple to use visual designer. How does it work? – You visually design the pipelines, and Prophecy generates clean Spark code with tests on git; then you visually schedule these pipelines on Airflow. You can observe your pipelines with built in metadata search and column level lineage. Finally, if you have existing workflows in AbInitio, Informatica or other ETL formats that you want to move to the cloud, you can import them automatically into Prophecy making them run productively on Spark. Create your free account today at dataengineeringpodcast.com/prophecy. The only thing worse than having bad data is not knowing that you have it. With Bigeye’s data observability platform, if there is an issue with your data or data pipelines you’ll know right away and can get it fixed before the business is impacted. Bigeye let’s data teams measure, improve, and communicate the quality of your data to company stakeholders. With complete API access, a user-friendly interface, and automated yet flexible alerting, you’ve got everything you need to establish and maintain trust in your data. Go to dataengineeringpodcast.com/bigeye today to sign up and start trusting your analyses. Your host is Tobias Macey and today I’m interviewing Matt Harrison about useful tips for using Pandas for data engineering projects Interview Introduction How did you get involved in the area of data management? What are the main tasks that you have seen Pandas used for in a data engineering context? What are some of the common mistakes that can lead to poor performance when scaling to large data sets? What are some of the utility features that you have found most helpful for data processing? One of the interesting add-ons to Pandas is its integration with Arrow. What are some of the considerations for how and when to use the Arrow capabilities vs. out-of-the-box Pandas? Pandas is a tool that spans data processing and data science. What are some of the ways that data engineers should think about writing their code to make it accessible to data scientists for supporting collaboration across data workflows? Pandas is often used for transformation logic. What are some of the ways that engineers should approach the design of their code to make it understandable and maintainable? How can data engineers support testing their transformations? There are a number of projects that aim to scale Pandas logic across cores and clusters. What are some of the considerations for when to use one of these tools, and how to select the proper framework? (e.g. Dask, Modin, Ray, etc.) What are some anti-patterns that engineers should guard against when using Pandas for data processing? What are the most interesting, innovative, or unexpected ways that you have seen Pandas used for data processing? When is Pandas the wrong choice for data processing? What are some of the projects related to Pandas that you are keeping an eye on? Contact Info @__mharrison__ on Twitter metasnake Effective Pandas Bundle (affiliate link with 20% discount code applied) Parting Question From your perspective, what is the biggest gap in the tooling or technology for data management today? Closing Announcements Thank you for listening! Don’t forget to check out our other show, Podcast.__init__ to learn about the Python language, its community, and the innovative ways it is being used. Visit the site to subscribe to the show, sign up for the mailing list, and read the show notes. If you’ve learned something or tried out a project from the show then tell us about it! Email [email protected]) with your story. To help other people find the show please leave a review on iTunes and tell your friends and co-workers Links Metasnake Snowflake Schema OLAP Panel Data NumPy Dask Podcast Episode Parquet Arrow Feather Zen of Python Joel Grus’ I Don’t Like Notebooks presentation Pandas Method Chaining Effective Pandas Book (affiliate link with 20% discount code applied) Podcast.__init__ Episode pytest Podcast.__init__ Episode Great Expectations Podcast Episode Hypothesis Podcast.__init__ Episode Papermill Podcast Episode Jupytext Koalas Modin Podcast.__init__ Episode Spark Ray Podcast.__init__ Episode Spark Pandas API Vaex Rapids Terality H2O H2O DataTable Fugue Ibis Multi-process Pandas PandaPy Polars Google Colab The intro and outro music is from The Hug by The Freak Fandango Orchestra / CC BY-SA Support Data Engineering Podcast