Hire And Scale Your Data Team With Intention
Data Engineering Podcast - A podcast by Tobias Macey - Duminică
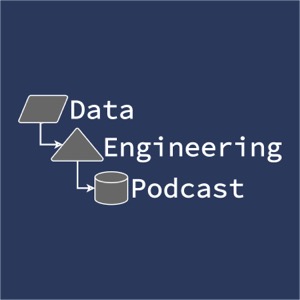
Categories:
Summary Building a well rounded and effective data team is an iterative process, and the first hire can set the stage for future success or failure. Trupti Natu has been the first data hire multiple times and gone through the process of building teams across the different stages of growth. In this episode she shares her thoughts and insights on how to be intentional about establishing your own data team. Announcements Hello and welcome to the Data Engineering Podcast, the show about modern data management When you’re ready to build your next pipeline, or want to test out the projects you hear about on the show, you’ll need somewhere to deploy it, so check out our friends at Linode. With their new managed database service you can launch a production ready MySQL, Postgres, or MongoDB cluster in minutes, with automated backups, 40 Gbps connections from your application hosts, and high throughput SSDs. Go to dataengineeringpodcast.com/linode today and get a $100 credit to launch a database, create a Kubernetes cluster, or take advantage of all of their other services. And don’t forget to thank them for their continued support of this show! Atlan is the metadata hub for your data ecosystem. Instead of locking all of that information into a new silo, unleash its transformative potential with Atlan’s active metadata capabilities. Push information about data freshness and quality to your business intelligence, automatically scale up and down your warehouse based on usage patterns, and let the bots answer those questions in Slack so that the humans can focus on delivering real value. Go to dataengineeringpodcast.com/atlan today to learn more about how you can take advantage of active metadata and escape the chaos. Atlan is the metadata hub for your data ecosystem. Instead of locking your metadata into a new silo, unleash its transformative potential with Atlan’s active metadata capabilities. Push information about data freshness and quality to your business intelligence, automatically scale up and down your warehouse based on usage patterns, and let the bots answer those questions in Slack so that the humans can focus on delivering real value. Go to dataengineeringpodcast.com/atlan today to learn more about how Atlan’s active metadata platform is helping pioneering data teams like Postman, Plaid, WeWork & Unilever achieve extraordinary things with metadata and escape the chaos. Modern data teams are dealing with a lot of complexity in their data pipelines and analytical code. Monitoring data quality, tracing incidents, and testing changes can be daunting and often takes hours to days or even weeks. By the time errors have made their way into production, it’s often too late and damage is done. Datafold built automated regression testing to help data and analytics engineers deal with data quality in their pull requests. Datafold shows how a change in SQL code affects your data, both on a statistical level and down to individual rows and values before it gets merged to production. No more shipping and praying, you can now know exactly what will change in your database! Datafold integrates with all major data warehouses as well as frameworks such as Airflow & dbt and seamlessly plugs into CI workflows. Visit dataengineeringpodcast.com/datafold today to book a demo with Datafold. Unstruk is the DataOps platform for your unstructured data. The options for ingesting, organizing, and curating unstructured files are complex, expensive, and bespoke. Unstruk Data is changing that equation with their platform approach to manage your unstructured assets. Built to handle all of your real-world data, from videos and images, to 3d point clouds and geospatial records, to industry specific file formats, Unstruk streamlines your workflow by converting human hours into machine minutes, and automatically alerting you to insights found in your dark data. Unstruk handles data versioning, lineage tracking, duplicate detection, consistency validation, as well as enrichment through sources including machine learning models, 3rd party data, and web APIs. Go to dataengineeringpodcast.com/unstruk today to transform your messy collection of unstructured data files into actionable assets that power your business. Your host is Tobias Macey and today I’m interviewing Trupti Natu about strategies for building your team, from the first data hire to post-acquisition Interview Introduction How did you get involved in the area of FinTech & Data Science (management)? How would you describe your overall career trajectory in data? Can you describe what your experience has been as a data professional at different stages of company growth? What are the traits that you look for in a first or second data hire at an organization? What are useful metrics for success to help gauge the effectiveness of hires at this early stage of data capabilities? What are the broad goals and projects that early data hires should be focused on? What are the indicators that you look for to determine when to scale the team? As you are building a team of data professionals, what are the organizational topologies that you have found most effective? (e.g. centralized vs. embedded data pros, etc.) What are the recruiting and screening/interviewing techniques that you have found most helpful given the relative scarcity of experienced data practitioners? What are the organizational and technical structures that are helpful to establish early in the organization’s data journey to reduce the onboarding time for new hires? Your background has primarily been in FinTech. How does the business domain influence the types of background and domain expertise that you look for? You recently went through an acquisition at the startup you were with. Can you describe the data-related projects that were required during the merger? What are the impedance mismatches that you have had to resolve in your data systems, moving from a fast-moving startup into a larger, more established organization? Being a FinTech company, what are some of the categories of regulatory considerations that you had to deal with during the integration process? What are the most interesting, unexpected, or challenging lessons that you have learned along your career journey? What are some of the pieces of advice that you wished you knew at the beginning of your career, and that you would like to share with others in that situation? Contact Info LinkedIn @truptinatu on Twitter Trupti is hiring for multiple product data science roles. Feel free to DM her on Twitter or LinkedIn to find out more Parting Question From your perspective, what is the biggest gap in the tooling or technology for data management today? Closing Announcements Thank you for listening! Don’t forget to check out our other shows. Podcast.__init__ covers the Python language, its community, and the innovative ways it is being used. The Machine Learning Podcast helps you go from idea to production with machine learning. Visit the site to subscribe to the show, sign up for the mailing list, and read the show notes. If you’ve learned something or tried out a project from the show then tell us about it! Email [email protected]) with your story. To help other people find the show please leave a review on iTunes and tell your friends and co-workers Links SumoLogic FinTech PII == Personally Identifiable Information The intro and outro music is from The Hug by The Freak Fandango Orchestra / CC BY-SA Support Data Engineering Podcast