Level Up Your Data Platform With Active Metadata
Data Engineering Podcast - A podcast by Tobias Macey - Duminică
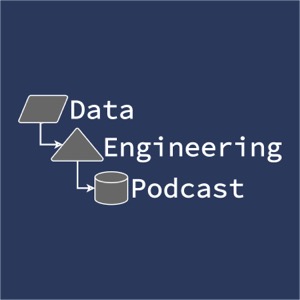
Categories:
Summary Metadata is the lifeblood of your data platform, providing information about what is happening in your systems. A variety of platforms have been developed to capture and analyze that information to great effect, but they are inherently limited in their utility due to their nature as storage systems. In order to level up their value a new trend of active metadata is being implemented, allowing use cases like keeping BI reports up to date, auto-scaling your warehouses, and automated data governance. In this episode Prukalpa Sankar joins the show to talk about the work she and her team at Atlan are doing to push this capability into the mainstream. Announcements Hello and welcome to the Data Engineering Podcast, the show about modern data management When you’re ready to build your next pipeline, or want to test out the projects you hear about on the show, you’ll need somewhere to deploy it, so check out our friends at Linode. With their new managed database service you can launch a production ready MySQL, Postgres, or MongoDB cluster in minutes, with automated backups, 40 Gbps connections from your application hosts, and high throughput SSDs. Go to dataengineeringpodcast.com/linode today and get a $100 credit to launch a database, create a Kubernetes cluster, or take advantage of all of their other services. And don’t forget to thank them for their continued support of this show! RudderStack helps you build a customer data platform on your warehouse or data lake. Instead of trapping data in a black box, they enable you to easily collect customer data from the entire stack and build an identity graph on your warehouse, giving you full visibility and control. Their SDKs make event streaming from any app or website easy, and their state-of-the-art reverse ETL pipelines enable you to send enriched data to any cloud tool. Sign up free… or just get the free t-shirt for being a listener of the Data Engineering Podcast at dataengineeringpodcast.com/rudder. Data teams are increasingly under pressure to deliver. According to a recent survey by Ascend.io, 95% in fact reported being at or over capacity. With 72% of data experts reporting demands on their team going up faster than they can hire, it’s no surprise they are increasingly turning to automation. In fact, while only 3.5% report having current investments in automation, 85% of data teams plan on investing in automation in the next 12 months. 85%!!! That’s where our friends at Ascend.io come in. The Ascend Data Automation Cloud provides a unified platform for data ingestion, transformation, orchestration, and observability. Ascend users love its declarative pipelines, powerful SDK, elegant UI, and extensible plug-in architecture, as well as its support for Python, SQL, Scala, and Java. Ascend automates workloads on Snowflake, Databricks, BigQuery, and open source Spark, and can be deployed in AWS, Azure, or GCP. Go to dataengineeringpodcast.com/ascend and sign up for a free trial. If you’re a data engineering podcast listener, you get credits worth $5,000 when you become a customer. Today’s episode is Sponsored by Prophecy.io – the low-code data engineering platform for the cloud. Prophecy provides an easy-to-use visual interface to design & deploy data pipelines on Apache Spark & Apache Airflow. Now all the data users can use software engineering best practices – git, tests and continuous deployment with a simple to use visual designer. How does it work? – You visually design the pipelines, and Prophecy generates clean Spark code with tests on git; then you visually schedule these pipelines on Airflow. You can observe your pipelines with built in metadata search and column level lineage. Finally, if you have existing workflows in AbInitio, Informatica or other ETL formats that you want to move to the cloud, you can import them automatically into Prophecy making them run productively on Spark. Create your free account today at dataengineeringpodcast.com/prophecy. Your host is Tobias Macey and today I’m interviewing Prukalpa Sankar about how data platforms can benefit from the idea of "active metadata" and the work that she and her team at Atlan are doing to make it a reality Interview Introduction How did you get involved in the area of data management? Can you describe what "active metadata" is and how it differs from the current approaches to metadata systems? What are some of the use cases that "active metadata" can enable for data producers and consumers? What are the points of friction that those users encounter in the current formulation of metadata systems? Central metadata systems/data catalogs came about as a solution to the challenge of integrating every data tool with every other data tool, giving a single place to integrate. What are the lessons that are being learned from the "modern data stack" that can be applied to centralized metadata? Can you describe the approach that you are taking at Atlan to enable the adoption of "active metadata"? What are the architectural capabilities that you had to build to power the outbound traffic flows? How are you addressing the N x M integration problem for pushing metadata into the necessary contexts at Atlan? What are the interfaces that are necessary for receiving systems to be able to make use of the metadata that is being delivered? How does the type/category of metadata impact the type of integration that is necessary? What are some of the automation possibilities that metadata activation offers for data teams? What are the cases where you still need a human in the loop? What are the most interesting, innovative, or unexpected ways that you have seen active metadata capabilities used? What are the most interesting, unexpected, or challenging lessons that you have learned while working on activating metadata for your users? When is an active approach to metadata the wrong choice? What do you have planned for the future of Atlan and active metadata? Contact Info LinkedIn @prukalpa on Twitter Parting Question From your perspective, what is the biggest gap in the tooling or technology for data management today? Closing Announcements Thank you for listening! Don’t forget to check out our other shows. Podcast.__init__ covers the Python language, its community, and the innovative ways it is being used. The Machine Learning Podcast helps you go from idea to production with machine learning. Visit the site to subscribe to the show, sign up for the mailing list, and read the show notes. If you’ve learned something or tried out a project from the show then tell us about it! Email [email protected]) with your story. To help other people find the show please leave a review on Apple Podcasts and tell your friends and co-workers Links Atlan What is Active Metadata? Segment Podcast Episode Zapier ArgoCD Kubernetes Wix AWS Lambda Modern Data Culture Blog Post The intro and outro music is from The Hug by The Freak Fandango Orchestra / CC BY-SA Support Data Engineering Podcast