Episode 65: AI knows biology. Or does it?
Data Science at Home - A podcast by Francesco Gadaleta
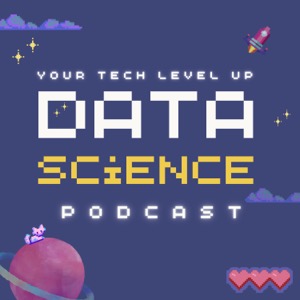
Categories:
The successes of deep learning for text analytics, also introduced in a recent post about sentiment analysis and published here are undeniable. Many other tasks in NLP have also benefitted from the superiority of deep learning methods over more traditional approaches. Such extraordinary results have also been possible due to the neural network approach to learn meaningful character and word embeddings, that is the representation space in which semantically similar objects are mapped to nearby vectors. All this is strictly related to a field one might initially find disconnected or off-topic: biology. Don't forget to subscribe to our Newsletter at amethix.com and get the latest updates in AI and machine learning. We do not spam. Promise! References [1] Rives A., et al., “Biological structure and function emerge from scaling unsupervised learning to 250 million protein sequences”, biorxiv, doi: https://doi.org/10.1101/622803 [2] Vaswani A., et al., “Attention is all you need”, Advances in neural information processing systems, pp. 5998–6008, 2017. [3] Bahdanau D., et al., “Neural machine translation by jointly learning to align and translate”, arXiv, http://arxiv.org/abs/1409.0473.