#113 A Deep Dive into Bayesian Stats, with Alex Andorra, ft. the Super Data Science Podcast
Learning Bayesian Statistics - A podcast by Alexandre Andorra - Miercuri
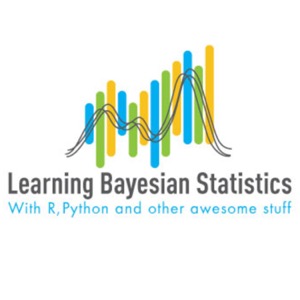
Categories:
Proudly sponsored by PyMC Labs, the Bayesian Consultancy. Book a call, or get in touch!My Intuitive Bayes Online Courses1:1 Mentorship with meOur theme music is « Good Bayesian », by Baba Brinkman (feat MC Lars and Mega Ran). Check out his awesome work!Visit our Patreon page to unlock exclusive Bayesian swag ;)Takeaways:Bayesian statistics is a powerful framework for handling complex problems, making use of prior knowledge, and excelling with limited data.Bayesian statistics provides a framework for updating beliefs and making predictions based on prior knowledge and observed data.Bayesian methods allow for the explicit incorporation of prior assumptions, which can provide structure and improve the reliability of the analysis.There are several Bayesian frameworks available, such as PyMC, Stan, and Bambi, each with its own strengths and features.PyMC is a powerful library for Bayesian modeling that allows for flexible and efficient computation.For beginners, it is recommended to start with introductory courses or resources that provide a step-by-step approach to learning Bayesian statistics.PyTensor leverages GPU acceleration and complex graph optimizations to improve the performance and scalability of Bayesian models.ArviZ is a library for post-modeling workflows in Bayesian statistics, providing tools for model diagnostics and result visualization.Gaussian processes are versatile non-parametric models that can be used for spatial and temporal data analysis in Bayesian statistics.Chapters:00:00 Introduction to Bayesian Statistics07:32 Advantages of Bayesian Methods16:22 Incorporating Priors in Models23:26 Modeling Causal Relationships30:03 Introduction to PyMC, Stan, and Bambi34:30 Choosing the Right Bayesian Framework39:20 Getting Started with Bayesian Statistics44:39 Understanding Bayesian Statistics and PyMC49:01 Leveraging PyTensor for Improved Performance and Scalability01:02:37 Exploring Post-Modeling Workflows with ArviZ01:08:30 The Power of Gaussian Processes in Bayesian ModelingThank you to my Patrons for making this episode possible!Yusuke Saito, Avi Bryant, Ero Carrera, Giuliano Cruz, Tim Gasser, James Wade, Tradd Salvo, William Benton, James Ahloy, Robin Taylor,, Chad Scherrer, Zwelithini Tunyiswa, Bertrand Wilden, James Thompson, Stephen Oates, Gian Luca Di Tanna,...