Vector Quantization for NN Compression with Julieta Martinez - #498
The TWIML AI Podcast (formerly This Week in Machine Learning & Artificial Intelligence) - A podcast by Sam Charrington - Luni
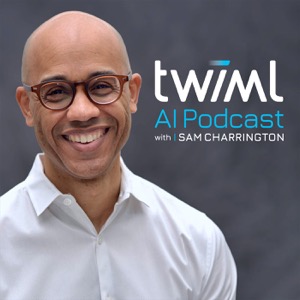
Categories:
Today we’re joined by Julieta Martinez, a senior research scientist at recently announced startup Waabi. Julieta was a keynote speaker at the recent LatinX in AI workshop at CVPR, and our conversation focuses on her talk “What do Large-Scale Visual Search and Neural Network Compression have in Common,” which shows that multiple ideas from large-scale visual search can be used to achieve state-of-the-art neural network compression. We explore the commonality between large databases and dealing with high dimensional, many-parameter neural networks, the advantages of using product quantization, and how that plays out when using it to compress a neural network. We also dig into another paper Julieta presented at the conference, Deep Multi-Task Learning for Joint Localization, Perception, and Prediction, which details an architecture that is able to reuse computation between the three tasks, and is thus able to correct localization errors efficiently. The complete show notes for this episode can be found at twimlai.com/go/498.